Data Science
Data science can predict the future
The car you drive is the product of one of the most complex logistical endeavours ever attempted. Tens of thousands of components and raw materials criss-cross the world to get to the right place at the right time for each vehicle to roll off the production line.
Holding spare stock costs money, so manufacturers prefer to rely on steady supplies of parts that are delivered exactly when they are needed. But the “just-in-time” model that underpins the automobile industry is vulnerable. Unexpected delays can have far-reaching and costly knock-on effects all the way along the supply chain.
The old saying that “for the want of a nail, a kingdom was lost” resonates in an industry where the want of a container of ball bearings can do serious damage to an automaker’s bottom line. But what if the company shipping that container knew that the usually reliable route would take too long this time, and automatically switched it to a quicker alternative?
Any tool that can predict when and where delays might happen, and work out ways to avoid them, will be extremely valuable. People cannot take into account every single one of the extraordinary number of variables affecting a sophisticated supply chain. Data science can.
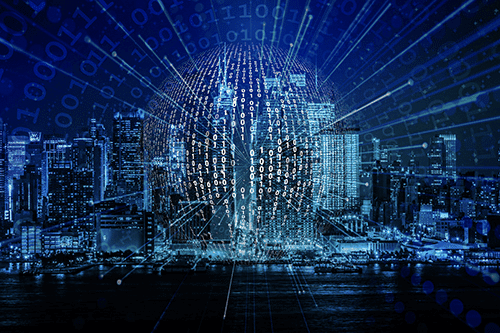
What is data science?
Data science involves analyzing information from the past and present, and using it to predict the future. And it isn’t new. Individuals and companies have long used ledgers, spreadsheets and computers to identify trends and spot potential problems and opportunities.
But the digital revolution is allowing data science to grow beyond anything seen before. Technologies such as the Internet of Things (IoT) are providing unprecedented quantities of data and track goods in real time as they move along the supply chain. Blockchain allows companies to trust that the data they are using (for example that a product has left a warehouse) is genuine and secure. Robotic Process Automation, or RPA, is eliminating errors from the data, guaranteeing its accuracy.
The result is data that gives a very detailed picture of the world. Using machine learning, computers can sift the information, looking for patterns. When a pattern is repeated often enough, it can be used as a guide to what is likely to happen in the future.
Why does it matter?
Uncertainty is damaging to every part of a supply chain. But for shipping companies themselves it can be fatal. Competitive pricing depends on correct estimates of the costs incurred. If you estimate too high, you risk being outbid. If you estimate too low, you will lose money on the delivery.
Reputation is extremely important to shipping companies. Customers rely on the promises we make in order to run their businesses. If those promises are broken, the crucial bond of trust is weakened. As supply chains become ever more sophisticated, data science will be essential for accurate pricing and ensuring that the promises made will be kept.
Although cost and speed are the decisive factors in many deliveries, other considerations are becoming more important too. Political and economic volatility make the security of a supply chain ever more important. An increased awareness of the environmental cost of transporting goods means that emissions and carbon footprint are becoming key factors in deciding routes.
Data science allows shipping companies to account for new pieces of information, such as a change in a security situation or new environmental regulation, much more quickly. The same reaction speed also means new markets and routes can be evaluated sooner.
Opportunities…
Knowing what the optimal route will be is one thing. Acting on that information is another. This is where artificial intelligence (AI) can play a role.
AI is the use of computers to react to data in the same way a human would. If a warehouse manager knows that a spilled load has caused a logjam at the site entrance, he might want to alert incoming trucks to postpone their collection, and warn the next link in the chain to expect a delay. But will he have the time to call each driver while he’s sorting out the spill?
AI systems can be programmed to immediately alert all the relevant links in the chain. A truck that was due to collect from the warehouse can be diverted to another delivery until the site has been cleared. The aircraft that would be waiting for its consignment can be loaded with an alternative cargo.
AI could also work out a new route for the delayed consignment that falls within tailored parameters. When does the delivery actually need to be at its final destination? Is it a perishable good that means speed is paramount? Or is a low carbon cost the most important factor?
The efficiency dividend of this kind of AI could be enormous. Avoiding bottlenecks in real time will ultimately drive down the cost, improve the speed, and mitigate the environmental implications of the entire logistics and supply industry.
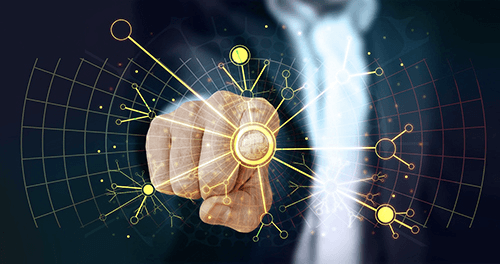
…and challenges
To make the kind of AI described above possible demands a high level of integration and interoperability. Data from multiple sources needs to be stored in a way that is accessible to all the relevant parties. This requires common standards and procedures so that different systems can effectively talk to each other.
The question of data ownership is controversial, and has not yet been resolved. Who, if anyone, can own the data? Who decides who can use it, and how? For example, take the case of tracking a shirt with an embedded IoT device from factory to retailer. Is the tracking data owned by the owner of the shirt? When does ownership of the shirt switch? Or does the data belong to the shipping company that is actually doing the tracking? Can the shipper use it to create proprietary business models? What happens to the data if the factory switches shipping companies? And whose rules apply in trade routes that span multiple countries?
These questions can only be answered as part of a broader global discussion. In the meantime, companies should ensure that they are compliant with data regulations that apply to any country jurisdiction they do business with. The EU’s GDPR laws are increasingly being seen as a gold standard that other countries will follow.
The final challenge is how to ensure AI responds in the right way. It is possible for computers to find patterns in data that are circumstantial, and have no predictive value at all. That means AI systems need to be monitored and evaluated.
Where now?
Data science is already playing a leading role in designing, managing and monitoring supply chains for bigger companies. Cloud computing is quickly making the technology cheaper and accessible to all. Shipping companies like Agility are already integrating advanced machine learning and AI into end-to-end services that are affordable for any customer, for example with our Shipa brand.
The data science revolution isn’t coming – it’s here already, and everyone can take part.
What we are learning
The greatest impact we’re seeing so far is in risk management. Until now, shippers have been using data to manage their “exceptions” – those instances when something in the supply chain goes wrong.
The next frontier is predictive analytics that allows shippers to address problems before they occur. That includes demand forecasting based on social media trends, consumption figures and other data to remove spikes and troughs in the supply chain and eliminate inventory bottlenecks.